Decentralizing DNA Data: Exploring Blockchain Solutions in a new Genomic Paradigm
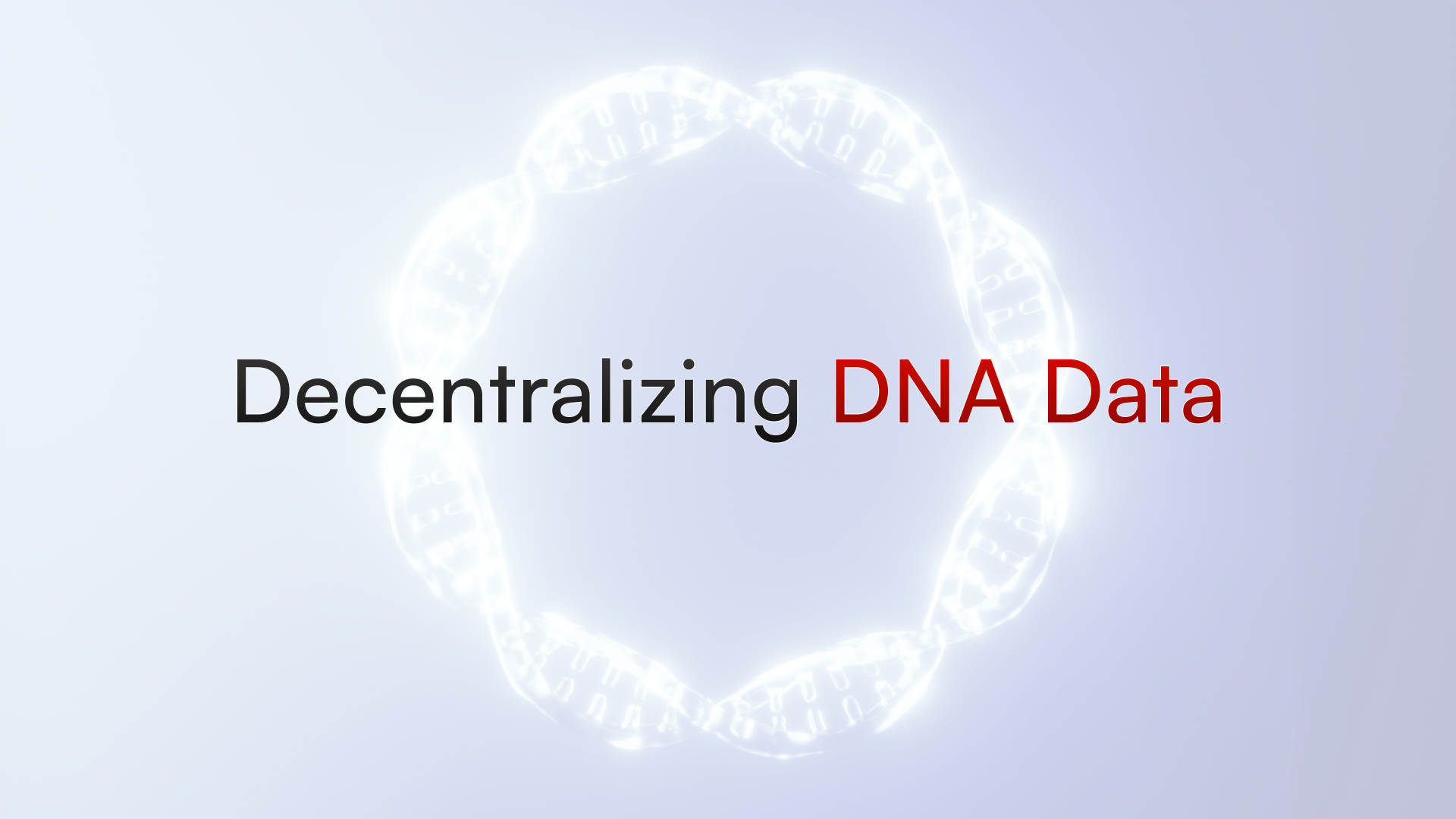
Genomic data has been referred to as “the new gold” by ex-23andMe CEO Anne Wojcicki. This article will explore relevant technical building blocks that could be incorporated into a next-gen genomic data platform to illustrate how these technical pieces could come together to serve users.
Flaws in Web2 Approaches to Health and Genomic Data
At its peak, 23andMe was the flagship name in direct-to-consumer genetics testing, accumulating around 15M individual samples. This collection represents one of the largest private repositories of human genetic information ever assembled, with vast implications for healthcare, drug development, and precision medicine. It promised us personalized health insights, ancestry tracing, and eventually, a new age of precision medicine with this treasure trove of human data. There are certain vulnerabilities and flaws ,however, that a blockchain based approach solves for that simply can’t be matched in a Web2 data storage environment. These flaws include:
Security Concerns Data breaches and exploits where access is centralized are ripe for attack. (For example, the Change Healthcare ransomware attack in 2024, and Oracle’s health data breaches in 2025). 23andMe’s major data breach in 2023 exposed the fundamental vulnerability of housing sensitive genomic data in centralized silos with a single point of failure.
Lack of Incentive Alignment In traditional Web2 economic models, if you aren’t paying, you are the product. Platforms like Epic and Cerner dominate the health data industry through enormous silos of information, sticky provider relationships, and low interoperability. Companies like 23andMe generate hundreds of millions in revenue from this data, monetizing it through partnerships with third parties, such as pharma and biotech. None of that value is shared with the very people who pay to provide it.
Lack of Composability Because of the inherently proprietary nature of data storage and transfer in Web2, little focus is placed on vertical integrations or other opportunities to build on top of datasets are missed. Breakthroughs could have been made in under-researched areas, e.g. women’s health and rare diseases. However, there was no way for developers, researchers, or startups to utilise this resource. The result: limited innovation, low utility, and a missed opportunity to create a thriving ecosystem.
By embracing a blockchain-based model for genomic data, security, privacy, and ethical monetization can be ensured, all while sidestepping some of the pitfalls that doomed legacy models. Blockchain technology has the potential to directly address each of these flaws: decentralization eliminates single points of failure; smart contracts ensure users get compensated for their data; and an open architecture enables innovation from developers worldwide.
To create a more secure, user-controlled genomic data platform, we need specific blockchain capabilities that can be used to build a new gold standard in user data management, learning from previous failures. Here are some of the key potential building blocks that could make this possible, each solving a critical problem that a centralized model couldn't overcome:
Blockchain Solutions
Onchain Record Keeping
Consent isn’t a checkbox, it’s the cornerstone of any ethical genomic ecosystem. By using smart contracts, we can verifiably ensure that every piece of data is used only with explicit permission. In this model, users are opted out by default; genomic data is only activated when users knowingly and explicitly opt in.
Onchain record keeping would rely on a smart contract that serves as a publicly auditable and verifiable papertrail for anyone to refer to. When a user opts in to share their genomic data, their decision is recorded via a transaction on the blockchain, linking their anonymized wallet address to a reference hash of an offchain anonymized data record.
This framework would provide transparency by allowing any verified participant to inspect the ledger. It would also facilitate automated processes such as reward distribution. When data is used, smart contracts could autonomously calculate and distribute rewards to users who have opted in, creating an economic incentive aligned with user privacy and consent.
Zero-Knowledge Proofs (ZKPs)
Zero Knowledge Proofs (ZKPs) allow one party to prove something is true without revealing any additional information. Imagine being able to prove that you're old enough to enter a bar without showing your exact birthdate. For genomic data, ZKPs solve critical privacy challenges, for example, a research team that receives a batch of genomic data and wants to verify certain characteristics of that data without exposing the sensitive data itself. Using ZKPs, these researchers could prove that all of the data meets some underlying requirement, such as the data belonging to people with pancreatic cancer, without exposing the complete individual records.
Decentralized Digital Identity schemes (DIDs) can be used alongside ZKPs to let said teams prove their credentials are valid and leave an auditable record onchain that verifies that the entity who accessed data met the legal requirements and were authorised to do so. In practice, when a research team requests access to data, they can generate a ZKP verifying the validity and compliance of their credentials, which a smart contract on the blockchain can quickly and securely verify to provide an immutable, privacy-preserving audit trail while leaving detailed records securely offchain.
By combining Merkle trees (a cryptographic data verification structure) with ZKPs, you can prove that every record in a dataset belongs to specific characteristics, for example: women aged 20–30, without revealing any sensitive details. Any specific characteristics important to research, legal, or ethical considerations could all be proven by writing custom circuits. For example, a circuit that each record has its gender encoded as “female” and a range proof that the age falls in the range 20 to 30. The circuit operates on confidential, committed inputs, and outputs a succinct proof that the entire dataset satisfies these criteria. With this approach, regulators or any interested party can verify the proof without gaining access to any underlying personal data, thus combining rigorous compliance with robust privacy. Alternatively a zkVM (Zero-Knowledge Virtual Machine) could allow for arbitrary proofs to be created without the need for custom circuits at the cost of the efficiency of generating said proofs.
Fully Homomorphic Encryption (FHE)
FHE is a cryptographic technique that allows for arbitrary computations to be performed on encrypted data without that data ever being visible. Though computationally expensive, FHE would allow for the processing of genomic data to be done without the data ever being exposed in a plain unencrypted form. When the genomic data is shipped to an external entity it would be provided in an encrypted form, and they would run their code against it without having access to the raw data.
To further enhance security and control over the sensitive data, a specialized key management strategy can be employed. In this model, while the data owner retains the primary decryption key for the genomic data, the buyer or processing entity is issued a restricted decryption key that allows them to decrypt only the computed results. This is possible through proxy reencryption. The external entity can now run their code and get their results without ever having the ability to directly access user data. This means that beyond securing individual data points, blockchain in association with cryptographic techniques like FHE can also transform how we learn from genomic data collectively.
Using FHE, a pharma company could run computations on genomic data to model things like the association between high glucose consumption and circulatory system illnesses, without needing access to the unencrypted underlying data itself. This allows for full privacy and security to be maintained, while still allowing the company to extract precise insights from the dataset.
Trusted Execution Environments (TEEs)
While ZKPs focus on proving facts without revealing data, Trusted Execution Environments (TEEs) provide a different approach to the same privacy problem: TEEs offer a hardware-based security approach to the processing of sensitive data without the need of fully homomorphic encryption (FHE). While FHE relies purely on cryptography to ensure data privacy, a TEE provides a secure hardware enclave to isolate and execute code in a trusted environment. This means that the data is unencrypted within the TEE but the data is still protected, and with a lower computational overhead than FHE. With a TEE, a company or researcher can provide their code to run in the TEE, have it audited prior to execution, and then the subsequent output exported back to the external entity.
This approach allows clients to run their analyses and view the results without ever having direct access to the underlying sensitive data, striking a balance between computational flexibility and data protection.
In practice, a TEE-based system could allow a researcher to securely build and run an algorithm that estimates an individuals chances of surviving a deadly disease based on their genomics data and a broader set of datapoints from previous survivors without exposing the sensitive data of the survivors themselves.
Federated Learning
Though not directly related to the storage or security of the data, blockchains introduce another opportunity for the use of the genomic data in the form of federated learning, with the blockchain being used to coordinate and attest to the learning process immutably. In federated learning protocols, multiple parties hold their own copy of the model and compute updates against the data they hold locally, that could mean allowing the health and other genomic data held offline to be included in a larger genomics training process without the data being directly shared. In the real world, this could mean that multiple independent biotech companies working on a similar vaccine, for example, could increase the amount of datapoints they have without exposing sensitive data to each other.
The blockchain can act as a messaging protocol recording updates and contributions to models linked to a verifiable entity, auditable by anyone. This not only prevents any single party from tampering with the learning process, but also creates a transparent record for rewarding contributions, enforcing compliance policies and tracing the provenance of each model improvement.
Product Example
Onchain Data Marketplaces
Having explored these technical building blocks individually, from secure record-keeping to privacy-preserving computation, we can now examine how they might work together in practice to create valuable real-world applications that directly address traditional Web2 shortcomings.
Utilizing the privacy of ZKPs, FHE or TEEs, and the transparent record-keeping described in this article, DNA data could be decentralized: imagine a true marketplace for genomic data that operates 24/7 with instant settlement and no unnecessary middlemen. This marketplace could align incentives between data providers and users, preserve privacy and security, and reduce overhead costs.Verifiable proofs could be used to prove that a participant meets the requirements to take part in an auction onchain, such as meeting any legal requirements and having been vetted by a trusted third party offchain, making it possible to maintain compliance on the data privacy side. On the user side, this would allow for seamless, efficient data monetization where users can choose if and who they sell their data to rather than having a third party do it on their behalf. In practice, this could look very similar to the onchain marketplaces of today, with advanced KYC and security controls gating access and ensuring compliance.
Here’s What an Onchain Marketplace for Genomic Data Could Look Like in Practice:
Supply Side
- Users upload their data: this could be genomics, wearables, biosamples, or imaging data. An aggregation of personal health and wellness datapoints, in one streamlined, encrypted application.
- User ID is abstracted away via a DID and wallet.
- Data is encrypted and stored on the Sei blockchain via FHE, TEE and/or ZKPs.
- Users can opt in to license the data to demand side participants.
Demand Side
- Possible participants include: biotech firms, Contract Research Organizations (CROs), insurance underwriters and AI researchers.
- All of these parties interact via a compliant onchain health data exchange.
- Purchasing entities can run complex simulations, computation, and analysis on the underlying dataset without being exposed to the raw data.
- Compliance can be baked in from day one (such as HIPAA/SOC2 via trusted partners).
In this system, users are compensated for their valuable data, and can specify who they want to sell their data to and at what price. Unlike traditional models where users received no compensation while their data was sold for hundreds of millions of dollars, a blockchain based marketplace could ensure users receive direct payment for their genomic information, with transparent tracing of every transaction. This creates a virtuous cycle where users are incentivized to provide more, and even higher quality data, purchasers can get access to this high quality data, privacy and security is preserved, and the platform grows through genuine value creation. Furthermore, buyers are able to get the most competitive pricing for the data they acquire, and aren't required to buy the entire dataset to get the pieces that they need. Both sides win, and do so without incurring additional fees through middlemen.
Conclusion
We are at a watershed moment that reveals the limitations of centralized approaches to sensitive personal data. By implementing blockchain technologies that address these core flaws, we can create a new genomic data ecosystem with fundamentally different properties:
- True User Control: People control their data and explicitly authorize each use case.
- Fair Compensation: Value flows back to data providers through transparent mechanisms.
- Privacy by Design: Technical safeguards ensure data remains protected even during complex analysis.
- Open Innovation: Developers can build applications on top of this infrastructure without gatekeepers.
The technical building blocks described here aren't theoretical, they exist today and can be assembled into a platform on a highly performant blockchain like Sei to serve both individual privacy and scientific progress. Blockchain, and Decentralized Science, offer not just an incremental improvement but a paradigm shift in how we handle one of our most personal and valuable assets: our genetic code.